Projects
The projects under study are as follows.
Speech emotion recognition
Speech emotion recognition predicts the emotional state of a speaker based on the person's speech. It brings an additional element for creating more natural human computer interactions. Earlier studies on emotional recognition have been primarily based on handcrafted features and manual labels. With the advent of deep learning, there have been some efforts in applying the deep-network-based approach to the problem of emotion recognition. As deep learning automatically extracts salient features correlated to speaker emotion, it brings certain advantages over the handcrafted-feature-based methods. There are, however, some challenges in applying them to the emotion recognition problem, because data required for properly training deep networks are often lacking. Therefore, there is a need for a new deep-learning-based approach which can exploit available information from given speech signals to the maximum extent possible. Our proposed method, called ``Fusion-ConvBERT'', is a parallel fusion model consisting of bidirectional encoder representations from transformers and convolutional neural networks.
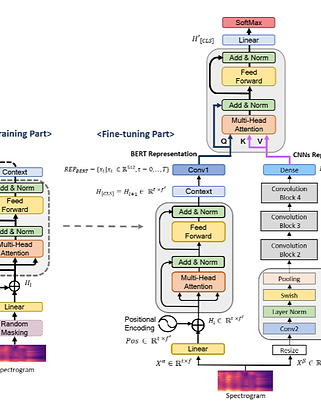
Multi-modal emotion recognition
Human communication includes rich emotional content, thus the development of multimodal emotion recognition plays an important role in communication between humans and computers. Because of the complex emotional characteristics of a speaker, emotional recognition remains a challenge, particularly in capturing emotional cues across a variety of modalities, such as speech, facial expressions, and language. Audio and visual cues are particularly vital for a human observer in understanding emotions. However, most previous work on emotion recognition has been based solely on linguistic information, which can overlook various forms of nonverbal information. In this paper, we present a new multimodal emotion recognition approach that improves the BERT model for emotion recognition by combining it with heterogeneous features based on language, audio, and visual modalities.
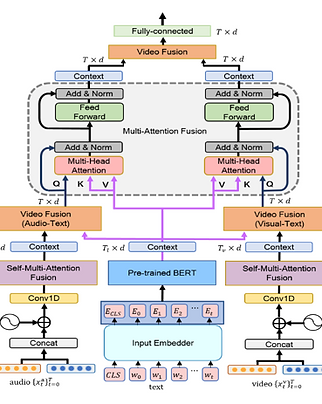
Speech recognition demo

Multimodal emotion recognition demo
